Last week AAPOR held it’s 69th annual conference in sunny (and hot) Anaheim California.

My biggest takeaway from this year’s conference is that AAPOR is a very healthy organization. AAPOR attendees were genuinely happy to be at the conference, enthusiastic about AAPOR and excited about the conference material. Many participants consider AAPOR their intellectual and professional home base and really relished the opportunity to be around kindred spirits (often socially awkward professionals who are genuinely excited about our niche). All of the presentations I saw firsthand or heard about were solid and dense, and the presenters were excited about their work and their findings. Membership, conference attendance, journal and conference submissions and volunteer participation are all quite strong.
At this point in time, the field of survey research is encountering a set of challenges. Nonresponse is a growing challenge, and other forms of data and analysis are increasingly en vogue. I was really excited to see that AAPOR members are greeting these challenges and others head on. For this particular write-up, I will focus on these two challenges. I hope that others will address some of the other main conference themes and add their notes and resources to those I’ve gathered below.
As survey nonresponse becomes more of a challenge, survey researchers are moving from traditional measures of response quality (e.g. response rates) to newer measures (e.g. nonresponse bias). Researchers are increasingly anchoring their discussions about survey quality within the Total Survey Error framework, which offers a contextual basis for understanding the problem more deeply. Instead of focusing on an across the board rise in response rates, researchers are strategizing their resources with the goal of reducing response bias. This includes understanding response propensity (who is likely not to respond to the survey? Who is most likely to drop out of a panel study? What are some of the barriers to survey participation?), looking for substantive measures that correlate with response propensity (e.g. Are small, rural private schools less likely to respond to a school survey? Are substance users less likely to respond to a survey about substance abuse?), and continuous monitoring of paradata during the collection period (e.g. developing differential strategies by disposition code, focusing the most successful interviewers on the most reluctant cases, or concentrating collection strategies where they are expected to be most effective). This area of strategizing emerged in AAPOR circles a few years ago with discussions of nonresponse propensity modeling, a process which is surely much more accessible than it sounds, but it has really evolved into a practical and useful tool that can help any size research shop increase survey quality and lower costs.
Another big takeaway for me was the volume of discussions and presentations that spoke to the fast-emerging world of data science and big data. Many people spoke of the importance of our voice in the realm of data science, particularly with our professional focus on understanding and mitigating errors in the research process. A few practitioners applied error frameworks to analyses of organic data, and some talks were based on analyses of organic data. This year AAPOR also sponsored a research hack to investigate the potential for Instagram as a research tool for Feed the Hungry. These discussions, presentations and activities made it clear that AAPOR will continue to have a strong voice in the changing research environment, and the task force reports and initiatives from both the membership and education committees reinforced AAPOR’s ability to be right on top of the many changes afoot. I’m eager to see AAPOR’s changing role take shape.
“If you had asked social scientists even 20 years ago what powers they dreamed of acquiring, they might have cited the capacity to track the behaviors, purchases, movements, interactions, and thoughts of whole cities of people, in real time.” – N.A. Christakis. 24 June 2011. New York Times, via Craig Hill (RTI)
AAPOR a very strong, well-loved organization and it is building a very strong future from a very solid foundation.
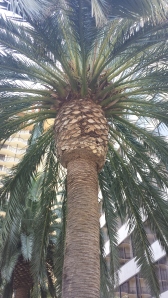
MORE DETAILED NOTES:
This conference is huge, so I could not possibly cover all of it on my own, so I will try to share my notes as well as the notes and resources I can collect from other attendees. If you have any materials to share, please send them to me! The more information I am able to collect here, the better a resource it will be for people interested in the AAPOR or the conference-
Patrick Ruffini assembled the tweets from the conference into this storify
Annie, the blogger behind LoveStats, had quite a few posts from the conference. I sat on a panel with Annie on the role of blogs in public opinion research (organized by Joe Murphy for the 68th annual AAPOR conference), and Annie blew me away by live-blogging the event from the stage! Clearly, she is the fastest blogger in the West and the East! Her posts from Anaheim included:
Your Significance Test Proves Nothing
Do panel companies manage their panels?
Gender bias among AAPOR presenters
What I hate about you AAPOR
How to correct scale distribution errors
What I like about you AAPOR
I poo poo on your significance tests
When is survey burden the fault of the responders?
How many survey contacts is enough?
My full notes are available here (please excuse any formatting irregularities). Unfortunately, they are not as extensive as I would have liked, because wifi and power were in short supply. I also wish I had settled into a better seat and covered some of the talks in greater detail, including Don Dillman’s talk, which was a real highlights of the conference!
I believe Rob Santos’ professional address will be available for viewing or listening soon, if it is not already available. He is a very eloquent speaker, and he made some really great points, so this will be well worth your time.